Image Deconvolution example for 511 keV with spacecraft attitude binning
updated on 2025-03-19
This notebook focuses on the image deconvolution with the spacecraft attitude (scatt) binning method. Using an older 511keV thin disk 3-month simulation data created for DC2, an example of the image analysis will be presented. If you have not run through 511keV-ScAtt-DataReduction.ipynb, please do that first as this analysis requires data that is binned slightly differently than the standard Galactic binning method shown in the DataIO tutorial.
[1]:
import logging
import sys
logger = logging.getLogger('cosipy')
logger.setLevel(logging.INFO)
logger.addHandler(logging.StreamHandler(sys.stdout))
[2]:
from histpy import Histogram, HealpixAxis, Axis, Axes
from mhealpy import HealpixMap
from astropy.coordinates import SkyCoord, cartesian_to_spherical, Galactic
from cosipy.response import FullDetectorResponse
from cosipy.spacecraftfile import SpacecraftFile
from cosipy.ts_map.TSMap import TSMap
from cosipy.data_io import UnBinnedData, BinnedData
from cosipy.image_deconvolution import SpacecraftAttitudeExposureTable, CoordsysConversionMatrix, DataIF_COSI_DC2, ImageDeconvolution
# cosipy uses astropy units
import astropy.units as u
from astropy.units import Quantity
from astropy.coordinates import SkyCoord
from astropy.time import Time
from astropy.table import Table
from astropy.io import fits
from scoords import Attitude, SpacecraftFrame
#3ML is needed for spectral modeling
from threeML import *
from astromodels import Band
#Other standard libraries
import numpy as np
import matplotlib.pyplot as plt
from matplotlib.gridspec import GridSpec
import healpy as hp
from tqdm.autonotebook import tqdm
%matplotlib inline
22:06:23 WARNING The naima package is not available. Models that depend on it will not be functions.py:48 available
WARNING The GSL library or the pygsl wrapper cannot be loaded. Models that depend on it functions.py:69 will not be available.
/Users/ckierans/Software/COSItools/COSItools/python-env/lib/python3.10/site-packages/numba-0.58.0-py3.10-macosx-14-arm64.egg/numba/core/decorators.py:262: NumbaDeprecationWarning: numba.generated_jit is deprecated. Please see the documentation at: https://numba.readthedocs.io/en/stable/reference/deprecation.html#deprecation-of-generated-jit for more information and advice on a suitable replacement.
warnings.warn(msg, NumbaDeprecationWarning)
WARNING The ebltable package is not available. Models that depend on it will not be absorption.py:33 available
/Users/ckierans/Software/COSItools/COSItools/python-env/lib/python3.10/site-packages/numba-0.58.0-py3.10-macosx-14-arm64.egg/numba/core/decorators.py:262: NumbaDeprecationWarning: numba.generated_jit is deprecated. Please see the documentation at: https://numba.readthedocs.io/en/stable/reference/deprecation.html#deprecation-of-generated-jit for more information and advice on a suitable replacement.
warnings.warn(msg, NumbaDeprecationWarning)
INFO Starting 3ML! __init__.py:39
WARNING WARNINGs here are NOT errors __init__.py:40
WARNING but are inform you about optional packages that can be installed __init__.py:41
WARNING to disable these messages, turn off start_warning in your config file __init__.py:44
22:06:23 WARNING ROOT minimizer not available minimization.py:1345
WARNING Multinest minimizer not available minimization.py:1357
WARNING PyGMO is not available minimization.py:1369
WARNING The cthreeML package is not installed. You will not be able to use plugins which __init__.py:94 require the C/C++ interface (currently HAWC)
WARNING Could not import plugin HAWCLike.py. Do you have the relative instrument __init__.py:144 software installed and configured?
WARNING Could not import plugin FermiLATLike.py. Do you have the relative instrument __init__.py:144 software installed and configured?
WARNING No fermitools installed lat_transient_builder.py:44
WARNING Env. variable OMP_NUM_THREADS is not set. Please set it to 1 for optimal __init__.py:387 performances in 3ML
WARNING Env. variable MKL_NUM_THREADS is not set. Please set it to 1 for optimal __init__.py:387 performances in 3ML
WARNING Env. variable NUMEXPR_NUM_THREADS is not set. Please set it to 1 for optimal __init__.py:387 performances in 3ML
0. Files needed for this notebook
You should have used the 511keV-ScAtt-DataReduction.ipynb notebook to download and bin the data sets needed for this notebook. Specicially, you now should have:
511keV_scatt_binning_DC2_bkg.hdf5
511keV_scatt_binning_DC2_event.hdf5
ccm.hdf5
From docs/tutorials/image_deconvolution/511keV/ScAttBinning, you will also need:
inputs_511keV.yaml
imagedeconvolution_parfile_scatt_511keV.yml
1. Read the response matrix
please modify “path_data” corresponding to your environment.
[ ]:
path_data = "/path/to/data/"
[4]:
response_path = path_data + "SMEXv12.511keV.HEALPixO4.binnedimaging.imagingresponse.nonsparse_nside16.area.h5"
response = FullDetectorResponse.open(response_path)
[5]:
response
[5]:
FILENAME: '/Users/ckierans/Software/COSItools/COSItools/cosipy/docs/tutorials/image_deconvolution/511keV/ScAttBinning/SMEXv12.511keV.HEALPixO4.binnedimaging.imagingresponse.nonsparse_nside16.area.h5'
AXES:
NuLambda:
DESCRIPTION: 'Location of the simulated source in the spacecraft coordinates'
TYPE: 'healpix'
NPIX: 3072
NSIDE: 16
SCHEME: 'RING'
Ei:
DESCRIPTION: 'Initial simulated energy'
TYPE: 'log'
UNIT: 'keV'
NBINS: 1
EDGES: [509.0 keV, 513.0 keV]
Em:
DESCRIPTION: 'Measured energy'
TYPE: 'log'
UNIT: 'keV'
NBINS: 1
EDGES: [509.0 keV, 513.0 keV]
Phi:
DESCRIPTION: 'Compton angle'
TYPE: 'linear'
UNIT: 'deg'
NBINS: 60
EDGES: [0.0 deg, 3.0 deg, 6.0 deg, 9.0 deg, 12.0 deg, 15.0 deg, 18.0 deg, 21.0 deg, 24.0 deg, 27.0 deg, 30.0 deg, 33.0 deg, 36.0 deg, 39.0 deg, 42.0 deg, 45.0 deg, 48.0 deg, 51.0 deg, 54.0 deg, 57.0 deg, 60.0 deg, 63.0 deg, 66.0 deg, 69.0 deg, 72.0 deg, 75.0 deg, 78.0 deg, 81.0 deg, 84.0 deg, 87.0 deg, 90.0 deg, 93.0 deg, 96.0 deg, 99.0 deg, 102.0 deg, 105.0 deg, 108.0 deg, 111.0 deg, 114.0 deg, 117.0 deg, 120.0 deg, 123.0 deg, 126.0 deg, 129.0 deg, 132.0 deg, 135.0 deg, 138.0 deg, 141.0 deg, 144.0 deg, 147.0 deg, 150.0 deg, 153.0 deg, 156.0 deg, 159.0 deg, 162.0 deg, 165.0 deg, 168.0 deg, 171.0 deg, 174.0 deg, 177.0 deg, 180.0 deg]
PsiChi:
DESCRIPTION: 'Location in the Compton Data Space'
TYPE: 'healpix'
NPIX: 3072
NSIDE: 16
SCHEME: 'RING'
2. Read binned 511keV binned files (source and background)
[6]:
%%time
# background
data_bkg = BinnedData("inputs_511keV.yaml")
data_bkg.load_binned_data_from_hdf5("511keV_dc2_scatt_bkg.hdf5")
## signal + background
data_511keV = BinnedData("inputs_511keV.yaml")
data_511keV.load_binned_data_from_hdf5("511keV_dc2_scatt_event.hdf5")
CPU times: user 117 ms, sys: 671 ms, total: 788 ms
Wall time: 809 ms
3. Load the coordsys conversion matrix
[7]:
%%time
ccm = CoordsysConversionMatrix.open("ccm_dc2.hdf5")
CPU times: user 1.21 s, sys: 64 ms, total: 1.28 s
Wall time: 1.28 s
4. Imaging deconvolution
Brief overview of the image deconvolution
Basically, we have to maximize the following likelihood function
\(X_i\): detected counts at \(i\)-th bin ( \(i\) : index of the Compton Data Space)
\(\epsilon_i = \sum_j R_{ij} \lambda_j + b_i\) : expected counts ( \(j\) : index of the model space)
\(\lambda_j\) : the model map (basically gamma-ray flux at \(j\)-th pixel)
\(b_i\) : the background at \(i\)-th bin
\(R_{ij}\) : the response matrix
Since we have to optimize the flux in each pixel, and the number of parameters is large, we adopt an iterative approach to find a solution of the above equation. The simplest one is the ML-EM (Maximum Likelihood Expectation Maximization) algorithm. It is also known as the Richardson-Lucy algorithm.
We refer to \(\delta \lambda_{j}^{k}\) as the delta map.
As for now, the two improved algorithms are implemented in COSIpy.
Accelerated ML-EM algorithm (Knoedlseder+99)
Practically, in order not to accelerate the algorithm excessively, we set the maximum value of \(\alpha\) (\(\alpha_{\mathrm{max}}\)). Then, \(\alpha\) is calculated as:
Noise damping using gaussian smoothing (Knoedlseder+05, Siegert+20)
\(\left[ ... \right]_{\mathrm{gauss}}\) means that the differential image is smoothed by a gaussian filter.
4-1. Prepare DataInterface containing all necessary datasets
[8]:
%%time
data_interface = DataIF_COSI_DC2.load(name = "511keV",
event_binned_data = data_511keV.binned_data,
dict_bkg_binned_data = {"albedo": data_bkg.binned_data},
rsp = response,
coordsys_conv_matrix=ccm,
is_miniDC2_format=False)
Loading the response matrix onto your computer memory...
Finished
... checking the axis ScAtt of the event and background files...
--> pass (edges)
... checking the axis Em of the event and background files...
--> pass (edges)
... checking the axis Phi of the event and background files...
--> pass (edges)
... checking the axis PsiChi of the event and background files...
--> pass (edges)
...checking the axis Em of the event and response files...
--> pass (edges)
...checking the axis Phi of the event and response files...
--> pass (edges)
...checking the axis PsiChi of the event and response files...
--> pass (edges)
The axes in the event and background files are redefined. Now they are consistent with those of the response file.
Calculating an exposure map...
Finished...
CPU times: user 6.67 s, sys: 1.49 s, total: 8.16 s
Wall time: 8.18 s
4-2. Initialize the instance of the image deconvolution class
First, we prepare an instance of the ImageDeconvolution class and then register the dataset and parameters for the deconvolution. After that, you can start the calculation.
please modify this parameter_filepath corresponding to your environment.
[9]:
parameter_filepath = "imagedeconvolution_parfile_scatt_511keV.yml"
[10]:
image_deconvolution = ImageDeconvolution()
# set data_interface to image_deconvolution
image_deconvolution.set_dataset([data_interface])
# set a parameter file for the image deconvolution
image_deconvolution.read_parameterfile(parameter_filepath)
Initialize image_deconvolution
In this process, a model map is defined following the input parameters, and it is initialized. Also, it prepares ancillary data for the image deconvolution, e.g., the expected counts with the initial model map, gaussian smoothing filter etc.
I describe parameters in the parameter file.
model_property
Name |
Unit |
Description |
Notes |
---|---|---|---|
coordinate |
str |
the coordinate system of the model map |
As for now, it must be ‘galactic’ |
nside |
int |
NSIDE of the model map |
it must be the same as NSIDE of ‘lb’ axis of the coordinate conversion matrix |
scheme |
str |
SCHEME of the model map |
As for now, it must be ‘ring’ |
energy_edges |
list of float [keV] |
The definition of the energy bins of the model map |
As for now, it must be the same as that of the response matrix |
model_initialization
Name |
Unit |
Description |
Notes |
---|---|---|---|
algorithm |
str |
the method name to initialize the model map |
As for now, only ‘flat’ can be used |
parameter_flat:values |
list of float [cm-2 s-1 sr-1] |
the list of photon fluxes for each energy band |
the length of the list should be the same as the length of “energy_edges” - 1 |
deconvolution
Name |
Unit |
Description |
Notes |
---|---|---|---|
algorithm |
str |
the name of the image deconvolution algorithm |
As for now, only ‘RL’ is supported |
parameter_RL:iteration |
int |
The maximum number of the iteration |
|
parameter_RL:acceleration |
bool |
whether the accelerated ML-EM algorithm (Knoedlseder+99) is used |
|
parameter_RL:alpha_max |
float |
the maximum value for the acceleration parameter |
|
parameter_RL:save_results_each_iteration |
bool |
whether an updated model map, detal map, likelihood etc. are saved at the end of each iteration |
|
parameter_RL:response_weighting |
bool |
whether a delta map is renormalized based on the exposure time on each pixel, namely \(w_j = (\sum_{i} R_{ij})^{\beta}\) (see Knoedlseder+05, Siegert+20) |
|
parameter_RL:response_weighting_index |
float |
\(\beta\) in the above equation |
|
parameter_RL:smoothing |
bool |
whether a Gaussian filter is used (see Knoedlseder+05, Siegert+20) |
|
parameter_RL:smoothing_FWHM |
float, degree |
the FWHM of the Gaussian in the filter |
|
parameter_RL:background_normalization_fitting |
bool |
whether the background normalization factor is optimized at each iteration |
As for now, the single background normalization factor is used in all of the bins |
parameter_RL:background_normalization_range |
list of float |
the range of the normalization factor |
should be positive |
[11]:
image_deconvolution.initialize()
#### Initialization Starts ####
<< Instantiating the model class AllSkyImage >>
---- parameters ----
coordinate: galactic
energy_edges:
unit: keV
value:
- 509.0
- 513.0
nside: 16
scheme: ring
unit: cm-2 s-1 sr-1
<< Setting initial values of the created model object >>
---- parameters ----
algorithm: flat
parameter:
unit: cm-2 s-1 sr-1
value:
- 1e-4
<< Registering the deconvolution algorithm >>
Gaussian filter with FWHM of 2.0 deg will be applied to delta images ...
---- parameters ----
algorithm: RL
parameter:
acceleration: true
alpha_max: 10.0
background_normalization_optimization: true
background_normalization_range:
albedo:
- 0.01
- 10.0
iteration_max: 10
response_weighting: true
response_weighting_index: 0.5
save_results: false
save_results_directory: ./results
smoothing: true
smoothing_FWHM:
unit: deg
value: 2.0
#### Initialization Finished ####
(You can change the parameters as follows)
Note that when you modify the parameters, do not forget to run “initialize” again!
[12]:
image_deconvolution.override_parameter("deconvolution:parameter:iteration_max = 30")
image_deconvolution.override_parameter("deconvolution:parameter:background_normalization_optimization = True")
image_deconvolution.override_parameter("deconvolution:parameter:alpha_max = 10")
image_deconvolution.override_parameter("deconvolution:parameter:smoothing_FWHM:value = 3.0")
image_deconvolution.initialize()
#### Initialization Starts ####
<< Instantiating the model class AllSkyImage >>
---- parameters ----
coordinate: galactic
energy_edges:
unit: keV
value:
- 509.0
- 513.0
nside: 16
scheme: ring
unit: cm-2 s-1 sr-1
<< Setting initial values of the created model object >>
---- parameters ----
algorithm: flat
parameter:
unit: cm-2 s-1 sr-1
value:
- 1e-4
<< Registering the deconvolution algorithm >>
Gaussian filter with FWHM of 3.0 deg will be applied to delta images ...
---- parameters ----
algorithm: RL
parameter:
acceleration: true
alpha_max: 10
background_normalization_optimization: true
background_normalization_range:
albedo:
- 0.01
- 10.0
iteration_max: 30
response_weighting: true
response_weighting_index: 0.5
save_results: false
save_results_directory: ./results
smoothing: true
smoothing_FWHM:
unit: deg
value: 3.0
#### Initialization Finished ####
4-3. Start the image deconvolution
On a MacBook Pro with M2 Max and 96 GB memory, it takes about 2.5 min for 30 iterations.
[13]:
%%time
image_deconvolution.run_deconvolution()
#### Image Deconvolution Starts ####
<< Initialization >>
The response weighting filter was calculated.
The expected count histograms were calculated with the initial model map.
## Iteration 1/30 ##
<< Pre-processing >>
<< E-step >>
<< M-step >>
<< Post-processing >>
WARNING RuntimeWarning: invalid value encountered in divide
<< Registering Result >>
alpha: 2.4783944324338596
background_normalization: {'albedo': 1.0003541905620614}
loglikelihood: [-1197056.4545480786]
<< Checking Stopping Criteria >>
--> Continue
## Iteration 2/30 ##
<< Pre-processing >>
<< E-step >>
<< M-step >>
<< Post-processing >>
WARNING RuntimeWarning: invalid value encountered in divide
<< Registering Result >>
alpha: 1.575421707005236
background_normalization: {'albedo': 0.9922607778263346}
loglikelihood: [-1148892.2494289856]
<< Checking Stopping Criteria >>
--> Continue
## Iteration 3/30 ##
<< Pre-processing >>
<< E-step >>
<< M-step >>
<< Post-processing >>
WARNING RuntimeWarning: invalid value encountered in divide
<< Registering Result >>
alpha: 10
background_normalization: {'albedo': 0.9989836398988254}
loglikelihood: [-1366763.493999292]
<< Checking Stopping Criteria >>
--> Continue
## Iteration 4/30 ##
<< Pre-processing >>
<< E-step >>
<< M-step >>
<< Post-processing >>
WARNING RuntimeWarning: invalid value encountered in divide
<< Registering Result >>
alpha: 1.0
background_normalization: {'albedo': 0.9807935141594855}
loglikelihood: [-1130201.6042120028]
<< Checking Stopping Criteria >>
--> Continue
## Iteration 5/30 ##
<< Pre-processing >>
<< E-step >>
<< M-step >>
<< Post-processing >>
WARNING RuntimeWarning: invalid value encountered in divide
<< Registering Result >>
alpha: 1.0
background_normalization: {'albedo': 0.9951524418084393}
loglikelihood: [-1124841.8752257773]
<< Checking Stopping Criteria >>
--> Continue
## Iteration 6/30 ##
<< Pre-processing >>
<< E-step >>
<< M-step >>
<< Post-processing >>
WARNING RuntimeWarning: invalid value encountered in divide
<< Registering Result >>
alpha: 1.0
background_normalization: {'albedo': 0.996710972292622}
loglikelihood: [-1123882.6965031484]
<< Checking Stopping Criteria >>
--> Continue
## Iteration 7/30 ##
<< Pre-processing >>
<< E-step >>
<< M-step >>
<< Post-processing >>
WARNING RuntimeWarning: invalid value encountered in divide
<< Registering Result >>
alpha: 1.0
background_normalization: {'albedo': 0.9968855622562821}
loglikelihood: [-1123152.1905212747]
<< Checking Stopping Criteria >>
--> Continue
## Iteration 8/30 ##
<< Pre-processing >>
<< E-step >>
<< M-step >>
<< Post-processing >>
WARNING RuntimeWarning: invalid value encountered in divide
<< Registering Result >>
alpha: 1.0
background_normalization: {'albedo': 0.9969083706965138}
loglikelihood: [-1122553.294395423]
<< Checking Stopping Criteria >>
--> Continue
## Iteration 9/30 ##
<< Pre-processing >>
<< E-step >>
<< M-step >>
<< Post-processing >>
WARNING RuntimeWarning: invalid value encountered in divide
<< Registering Result >>
alpha: 1.0
background_normalization: {'albedo': 0.9969134554559144}
loglikelihood: [-1122056.1156774329]
<< Checking Stopping Criteria >>
--> Continue
## Iteration 10/30 ##
<< Pre-processing >>
<< E-step >>
<< M-step >>
<< Post-processing >>
WARNING RuntimeWarning: invalid value encountered in divide
<< Registering Result >>
alpha: 1.0
background_normalization: {'albedo': 0.9969163516897256}
loglikelihood: [-1121639.152483511]
<< Checking Stopping Criteria >>
--> Continue
## Iteration 11/30 ##
<< Pre-processing >>
<< E-step >>
<< M-step >>
<< Post-processing >>
WARNING RuntimeWarning: invalid value encountered in divide
<< Registering Result >>
alpha: 1.0
background_normalization: {'albedo': 0.9969189620579678}
loglikelihood: [-1121286.2048804823]
<< Checking Stopping Criteria >>
--> Continue
## Iteration 12/30 ##
<< Pre-processing >>
<< E-step >>
<< M-step >>
<< Post-processing >>
WARNING RuntimeWarning: invalid value encountered in divide
<< Registering Result >>
alpha: 1.0
background_normalization: {'albedo': 0.9969215191961452}
loglikelihood: [-1120984.8976566715]
<< Checking Stopping Criteria >>
--> Continue
## Iteration 13/30 ##
<< Pre-processing >>
<< E-step >>
<< M-step >>
<< Post-processing >>
WARNING RuntimeWarning: invalid value encountered in divide
<< Registering Result >>
alpha: 1.0
background_normalization: {'albedo': 0.996924030389004}
loglikelihood: [-1120725.6564115596]
<< Checking Stopping Criteria >>
--> Continue
## Iteration 14/30 ##
<< Pre-processing >>
<< E-step >>
<< M-step >>
<< Post-processing >>
WARNING RuntimeWarning: invalid value encountered in divide
<< Registering Result >>
alpha: 1.0
background_normalization: {'albedo': 0.9969264790341887}
loglikelihood: [-1120500.9815390154]
<< Checking Stopping Criteria >>
--> Continue
## Iteration 15/30 ##
<< Pre-processing >>
<< E-step >>
<< M-step >>
<< Post-processing >>
WARNING RuntimeWarning: invalid value encountered in divide
<< Registering Result >>
alpha: 1.0
background_normalization: {'albedo': 0.9969288556146668}
loglikelihood: [-1120304.963459809]
<< Checking Stopping Criteria >>
--> Continue
## Iteration 16/30 ##
<< Pre-processing >>
<< E-step >>
<< M-step >>
<< Post-processing >>
WARNING RuntimeWarning: invalid value encountered in divide
<< Registering Result >>
alpha: 1.0
background_normalization: {'albedo': 0.9969311484060269}
loglikelihood: [-1120132.8961275548]
<< Checking Stopping Criteria >>
--> Continue
## Iteration 17/30 ##
<< Pre-processing >>
<< E-step >>
<< M-step >>
<< Post-processing >>
WARNING RuntimeWarning: invalid value encountered in divide
<< Registering Result >>
alpha: 1.0
background_normalization: {'albedo': 0.9969333516388736}
loglikelihood: [-1119980.969809744]
<< Checking Stopping Criteria >>
--> Continue
## Iteration 18/30 ##
<< Pre-processing >>
<< E-step >>
<< M-step >>
<< Post-processing >>
WARNING RuntimeWarning: invalid value encountered in divide
<< Registering Result >>
alpha: 1.0
background_normalization: {'albedo': 0.9969354690483336}
loglikelihood: [-1119846.1195404662]
<< Checking Stopping Criteria >>
--> Continue
## Iteration 19/30 ##
<< Pre-processing >>
<< E-step >>
<< M-step >>
<< Post-processing >>
WARNING RuntimeWarning: invalid value encountered in divide
<< Registering Result >>
alpha: 1.0
background_normalization: {'albedo': 0.9969374982210912}
loglikelihood: [-1119725.820978066]
<< Checking Stopping Criteria >>
--> Continue
## Iteration 20/30 ##
<< Pre-processing >>
<< E-step >>
<< M-step >>
<< Post-processing >>
WARNING RuntimeWarning: invalid value encountered in divide
<< Registering Result >>
alpha: 1.0
background_normalization: {'albedo': 0.9969394510025879}
loglikelihood: [-1119618.0199778753]
<< Checking Stopping Criteria >>
--> Continue
## Iteration 21/30 ##
<< Pre-processing >>
<< E-step >>
<< M-step >>
<< Post-processing >>
WARNING RuntimeWarning: invalid value encountered in divide
<< Registering Result >>
alpha: 1.0
background_normalization: {'albedo': 0.9969413216933766}
loglikelihood: [-1119520.9964885826]
<< Checking Stopping Criteria >>
--> Continue
## Iteration 22/30 ##
<< Pre-processing >>
<< E-step >>
<< M-step >>
WARNING RuntimeWarning: invalid value encountered in divide
<< Post-processing >>
<< Registering Result >>
alpha: 1.0
background_normalization: {'albedo': 0.9969431196567854}
loglikelihood: [-1119433.3289185578]
<< Checking Stopping Criteria >>
--> Continue
## Iteration 23/30 ##
<< Pre-processing >>
<< E-step >>
<< M-step >>
<< Post-processing >>
WARNING RuntimeWarning: invalid value encountered in divide
<< Registering Result >>
alpha: 1.0
background_normalization: {'albedo': 0.9969448441589266}
loglikelihood: [-1119353.8044356154]
<< Checking Stopping Criteria >>
--> Continue
## Iteration 24/30 ##
<< Pre-processing >>
<< E-step >>
<< M-step >>
<< Post-processing >>
WARNING RuntimeWarning: invalid value encountered in divide
<< Registering Result >>
alpha: 1.0
background_normalization: {'albedo': 0.9969465063444388}
loglikelihood: [-1119281.4278713942]
<< Checking Stopping Criteria >>
--> Continue
## Iteration 25/30 ##
<< Pre-processing >>
<< E-step >>
<< M-step >>
<< Post-processing >>
WARNING RuntimeWarning: invalid value encountered in divide
<< Registering Result >>
alpha: 1.0
background_normalization: {'albedo': 0.9969481006699753}
loglikelihood: [-1119215.3384681274]
<< Checking Stopping Criteria >>
--> Continue
## Iteration 26/30 ##
<< Pre-processing >>
<< E-step >>
<< M-step >>
<< Post-processing >>
WARNING RuntimeWarning: invalid value encountered in divide
<< Registering Result >>
alpha: 1.0
background_normalization: {'albedo': 0.9969496370088307}
loglikelihood: [-1119154.8018173785]
<< Checking Stopping Criteria >>
--> Continue
## Iteration 27/30 ##
<< Pre-processing >>
<< E-step >>
<< M-step >>
<< Post-processing >>
WARNING RuntimeWarning: invalid value encountered in divide
<< Registering Result >>
alpha: 1.0
background_normalization: {'albedo': 0.9969511182269674}
loglikelihood: [-1119099.190130895]
<< Checking Stopping Criteria >>
--> Continue
## Iteration 28/30 ##
<< Pre-processing >>
<< E-step >>
<< M-step >>
<< Post-processing >>
WARNING RuntimeWarning: invalid value encountered in divide
<< Registering Result >>
alpha: 1.0
background_normalization: {'albedo': 0.9969525475454646}
loglikelihood: [-1119047.9797920918]
<< Checking Stopping Criteria >>
--> Continue
## Iteration 29/30 ##
<< Pre-processing >>
<< E-step >>
<< M-step >>
<< Post-processing >>
WARNING RuntimeWarning: invalid value encountered in divide
<< Registering Result >>
alpha: 1.0
background_normalization: {'albedo': 0.9969539225343819}
loglikelihood: [-1119000.7175890533]
<< Checking Stopping Criteria >>
--> Continue
## Iteration 30/30 ##
<< Pre-processing >>
<< E-step >>
<< M-step >>
<< Post-processing >>
WARNING RuntimeWarning: invalid value encountered in divide
<< Registering Result >>
alpha: 1.0
background_normalization: {'albedo': 0.9969552449401047}
loglikelihood: [-1118956.9770636673]
<< Checking Stopping Criteria >>
--> Stop
<< Finalization >>
#### Image Deconvolution Finished ####
CPU times: user 18min 4s, sys: 3min 54s, total: 21min 58s
Wall time: 2min 41s
[14]:
image_deconvolution.results
[14]:
[{'iteration': 1,
'model': <cosipy.image_deconvolution.allskyimage.AllSkyImageModel at 0x4078c72e0>,
'delta_model': <cosipy.image_deconvolution.allskyimage.AllSkyImageModel at 0x4078c6860>,
'processed_delta_model': <cosipy.image_deconvolution.allskyimage.AllSkyImageModel at 0x4078c6b00>,
'background_normalization': {'albedo': 1.0003541905620614},
'alpha': <Quantity 2.47839443>,
'loglikelihood': [-1197056.4545480786]},
{'iteration': 2,
'model': <cosipy.image_deconvolution.allskyimage.AllSkyImageModel at 0x4078c4730>,
'delta_model': <cosipy.image_deconvolution.allskyimage.AllSkyImageModel at 0x4078c4a00>,
'processed_delta_model': <cosipy.image_deconvolution.allskyimage.AllSkyImageModel at 0x4078c4760>,
'background_normalization': {'albedo': 0.9922607778263346},
'alpha': <Quantity 1.57542171>,
'loglikelihood': [-1148892.2494289856]},
{'iteration': 3,
'model': <cosipy.image_deconvolution.allskyimage.AllSkyImageModel at 0x4078a42e0>,
'delta_model': <cosipy.image_deconvolution.allskyimage.AllSkyImageModel at 0x4078a76d0>,
'processed_delta_model': <cosipy.image_deconvolution.allskyimage.AllSkyImageModel at 0x4077e1cc0>,
'background_normalization': {'albedo': 0.9989836398988254},
'alpha': 10,
'loglikelihood': [-1366763.493999292]},
{'iteration': 4,
'model': <cosipy.image_deconvolution.allskyimage.AllSkyImageModel at 0x4078a4c10>,
'delta_model': <cosipy.image_deconvolution.allskyimage.AllSkyImageModel at 0x4078a4ca0>,
'processed_delta_model': <cosipy.image_deconvolution.allskyimage.AllSkyImageModel at 0x4078a5690>,
'background_normalization': {'albedo': 0.9807935141594855},
'alpha': 1.0,
'loglikelihood': [-1130201.6042120028]},
{'iteration': 5,
'model': <cosipy.image_deconvolution.allskyimage.AllSkyImageModel at 0x407833c70>,
'delta_model': <cosipy.image_deconvolution.allskyimage.AllSkyImageModel at 0x407833160>,
'processed_delta_model': <cosipy.image_deconvolution.allskyimage.AllSkyImageModel at 0x4078c6c20>,
'background_normalization': {'albedo': 0.9951524418084393},
'alpha': 1.0,
'loglikelihood': [-1124841.8752257773]},
{'iteration': 6,
'model': <cosipy.image_deconvolution.allskyimage.AllSkyImageModel at 0x407821390>,
'delta_model': <cosipy.image_deconvolution.allskyimage.AllSkyImageModel at 0x407823fa0>,
'processed_delta_model': <cosipy.image_deconvolution.allskyimage.AllSkyImageModel at 0x407821cc0>,
'background_normalization': {'albedo': 0.996710972292622},
'alpha': 1.0,
'loglikelihood': [-1123882.6965031484]},
{'iteration': 7,
'model': <cosipy.image_deconvolution.allskyimage.AllSkyImageModel at 0x4078a6a70>,
'delta_model': <cosipy.image_deconvolution.allskyimage.AllSkyImageModel at 0x4078a5270>,
'processed_delta_model': <cosipy.image_deconvolution.allskyimage.AllSkyImageModel at 0x4077e0760>,
'background_normalization': {'albedo': 0.9968855622562821},
'alpha': 1.0,
'loglikelihood': [-1123152.1905212747]},
{'iteration': 8,
'model': <cosipy.image_deconvolution.allskyimage.AllSkyImageModel at 0x4078117e0>,
'delta_model': <cosipy.image_deconvolution.allskyimage.AllSkyImageModel at 0x407813a30>,
'processed_delta_model': <cosipy.image_deconvolution.allskyimage.AllSkyImageModel at 0x40786b940>,
'background_normalization': {'albedo': 0.9969083706965138},
'alpha': 1.0,
'loglikelihood': [-1122553.294395423]},
{'iteration': 9,
'model': <cosipy.image_deconvolution.allskyimage.AllSkyImageModel at 0x4078129b0>,
'delta_model': <cosipy.image_deconvolution.allskyimage.AllSkyImageModel at 0x4078136a0>,
'processed_delta_model': <cosipy.image_deconvolution.allskyimage.AllSkyImageModel at 0x407812560>,
'background_normalization': {'albedo': 0.9969134554559144},
'alpha': 1.0,
'loglikelihood': [-1122056.1156774329]},
{'iteration': 10,
'model': <cosipy.image_deconvolution.allskyimage.AllSkyImageModel at 0x40786bdf0>,
'delta_model': <cosipy.image_deconvolution.allskyimage.AllSkyImageModel at 0x407810670>,
'processed_delta_model': <cosipy.image_deconvolution.allskyimage.AllSkyImageModel at 0x4078120e0>,
'background_normalization': {'albedo': 0.9969163516897256},
'alpha': 1.0,
'loglikelihood': [-1121639.152483511]},
{'iteration': 11,
'model': <cosipy.image_deconvolution.allskyimage.AllSkyImageModel at 0x40778bdc0>,
'delta_model': <cosipy.image_deconvolution.allskyimage.AllSkyImageModel at 0x40778ba90>,
'processed_delta_model': <cosipy.image_deconvolution.allskyimage.AllSkyImageModel at 0x40778bf10>,
'background_normalization': {'albedo': 0.9969189620579678},
'alpha': 1.0,
'loglikelihood': [-1121286.2048804823]},
{'iteration': 12,
'model': <cosipy.image_deconvolution.allskyimage.AllSkyImageModel at 0x40778a2f0>,
'delta_model': <cosipy.image_deconvolution.allskyimage.AllSkyImageModel at 0x407788d30>,
'processed_delta_model': <cosipy.image_deconvolution.allskyimage.AllSkyImageModel at 0x40768fdc0>,
'background_normalization': {'albedo': 0.9969215191961452},
'alpha': 1.0,
'loglikelihood': [-1120984.8976566715]},
{'iteration': 13,
'model': <cosipy.image_deconvolution.allskyimage.AllSkyImageModel at 0x40764c4f0>,
'delta_model': <cosipy.image_deconvolution.allskyimage.AllSkyImageModel at 0x40764ca30>,
'processed_delta_model': <cosipy.image_deconvolution.allskyimage.AllSkyImageModel at 0x40778a3e0>,
'background_normalization': {'albedo': 0.996924030389004},
'alpha': 1.0,
'loglikelihood': [-1120725.6564115596]},
{'iteration': 14,
'model': <cosipy.image_deconvolution.allskyimage.AllSkyImageModel at 0x40778a560>,
'delta_model': <cosipy.image_deconvolution.allskyimage.AllSkyImageModel at 0x40778a800>,
'processed_delta_model': <cosipy.image_deconvolution.allskyimage.AllSkyImageModel at 0x40778b1c0>,
'background_normalization': {'albedo': 0.9969264790341887},
'alpha': 1.0,
'loglikelihood': [-1120500.9815390154]},
{'iteration': 15,
'model': <cosipy.image_deconvolution.allskyimage.AllSkyImageModel at 0x407811f00>,
'delta_model': <cosipy.image_deconvolution.allskyimage.AllSkyImageModel at 0x407812b90>,
'processed_delta_model': <cosipy.image_deconvolution.allskyimage.AllSkyImageModel at 0x407812b00>,
'background_normalization': {'albedo': 0.9969288556146668},
'alpha': 1.0,
'loglikelihood': [-1120304.963459809]},
{'iteration': 16,
'model': <cosipy.image_deconvolution.allskyimage.AllSkyImageModel at 0x40778a890>,
'delta_model': <cosipy.image_deconvolution.allskyimage.AllSkyImageModel at 0x40778b5e0>,
'processed_delta_model': <cosipy.image_deconvolution.allskyimage.AllSkyImageModel at 0x40778b700>,
'background_normalization': {'albedo': 0.9969311484060269},
'alpha': 1.0,
'loglikelihood': [-1120132.8961275548]},
{'iteration': 17,
'model': <cosipy.image_deconvolution.allskyimage.AllSkyImageModel at 0x40764d3f0>,
'delta_model': <cosipy.image_deconvolution.allskyimage.AllSkyImageModel at 0x40764d5a0>,
'processed_delta_model': <cosipy.image_deconvolution.allskyimage.AllSkyImageModel at 0x40764d360>,
'background_normalization': {'albedo': 0.9969333516388736},
'alpha': 1.0,
'loglikelihood': [-1119980.969809744]},
{'iteration': 18,
'model': <cosipy.image_deconvolution.allskyimage.AllSkyImageModel at 0x407788a30>,
'delta_model': <cosipy.image_deconvolution.allskyimage.AllSkyImageModel at 0x4078c5a80>,
'processed_delta_model': <cosipy.image_deconvolution.allskyimage.AllSkyImageModel at 0x40786bac0>,
'background_normalization': {'albedo': 0.9969354690483336},
'alpha': 1.0,
'loglikelihood': [-1119846.1195404662]},
{'iteration': 19,
'model': <cosipy.image_deconvolution.allskyimage.AllSkyImageModel at 0x40764e950>,
'delta_model': <cosipy.image_deconvolution.allskyimage.AllSkyImageModel at 0x40764f4c0>,
'processed_delta_model': <cosipy.image_deconvolution.allskyimage.AllSkyImageModel at 0x40764dc60>,
'background_normalization': {'albedo': 0.9969374982210912},
'alpha': 1.0,
'loglikelihood': [-1119725.820978066]},
{'iteration': 20,
'model': <cosipy.image_deconvolution.allskyimage.AllSkyImageModel at 0x407823070>,
'delta_model': <cosipy.image_deconvolution.allskyimage.AllSkyImageModel at 0x407788f40>,
'processed_delta_model': <cosipy.image_deconvolution.allskyimage.AllSkyImageModel at 0x40778a860>,
'background_normalization': {'albedo': 0.9969394510025879},
'alpha': 1.0,
'loglikelihood': [-1119618.0199778753]},
{'iteration': 21,
'model': <cosipy.image_deconvolution.allskyimage.AllSkyImageModel at 0x4076352d0>,
'delta_model': <cosipy.image_deconvolution.allskyimage.AllSkyImageModel at 0x407634f10>,
'processed_delta_model': <cosipy.image_deconvolution.allskyimage.AllSkyImageModel at 0x4076362f0>,
'background_normalization': {'albedo': 0.9969413216933766},
'alpha': 1.0,
'loglikelihood': [-1119520.9964885826]},
{'iteration': 22,
'model': <cosipy.image_deconvolution.allskyimage.AllSkyImageModel at 0x407789750>,
'delta_model': <cosipy.image_deconvolution.allskyimage.AllSkyImageModel at 0x40778abf0>,
'processed_delta_model': <cosipy.image_deconvolution.allskyimage.AllSkyImageModel at 0x4077887c0>,
'background_normalization': {'albedo': 0.9969431196567854},
'alpha': 1.0,
'loglikelihood': [-1119433.3289185578]},
{'iteration': 23,
'model': <cosipy.image_deconvolution.allskyimage.AllSkyImageModel at 0x40764c700>,
'delta_model': <cosipy.image_deconvolution.allskyimage.AllSkyImageModel at 0x40764c2b0>,
'processed_delta_model': <cosipy.image_deconvolution.allskyimage.AllSkyImageModel at 0x40764f850>,
'background_normalization': {'albedo': 0.9969448441589266},
'alpha': 1.0,
'loglikelihood': [-1119353.8044356154]},
{'iteration': 24,
'model': <cosipy.image_deconvolution.allskyimage.AllSkyImageModel at 0x40764c040>,
'delta_model': <cosipy.image_deconvolution.allskyimage.AllSkyImageModel at 0x4078230d0>,
'processed_delta_model': <cosipy.image_deconvolution.allskyimage.AllSkyImageModel at 0x40764e800>,
'background_normalization': {'albedo': 0.9969465063444388},
'alpha': 1.0,
'loglikelihood': [-1119281.4278713942]},
{'iteration': 25,
'model': <cosipy.image_deconvolution.allskyimage.AllSkyImageModel at 0x4078690c0>,
'delta_model': <cosipy.image_deconvolution.allskyimage.AllSkyImageModel at 0x40786a5f0>,
'processed_delta_model': <cosipy.image_deconvolution.allskyimage.AllSkyImageModel at 0x40786b760>,
'background_normalization': {'albedo': 0.9969481006699753},
'alpha': 1.0,
'loglikelihood': [-1119215.3384681274]},
{'iteration': 26,
'model': <cosipy.image_deconvolution.allskyimage.AllSkyImageModel at 0x407637820>,
'delta_model': <cosipy.image_deconvolution.allskyimage.AllSkyImageModel at 0x4076363b0>,
'processed_delta_model': <cosipy.image_deconvolution.allskyimage.AllSkyImageModel at 0x4076379d0>,
'background_normalization': {'albedo': 0.9969496370088307},
'alpha': 1.0,
'loglikelihood': [-1119154.8018173785]},
{'iteration': 27,
'model': <cosipy.image_deconvolution.allskyimage.AllSkyImageModel at 0x407634be0>,
'delta_model': <cosipy.image_deconvolution.allskyimage.AllSkyImageModel at 0x407635780>,
'processed_delta_model': <cosipy.image_deconvolution.allskyimage.AllSkyImageModel at 0x407636680>,
'background_normalization': {'albedo': 0.9969511182269674},
'alpha': 1.0,
'loglikelihood': [-1119099.190130895]},
{'iteration': 28,
'model': <cosipy.image_deconvolution.allskyimage.AllSkyImageModel at 0x407634e80>,
'delta_model': <cosipy.image_deconvolution.allskyimage.AllSkyImageModel at 0x407636ef0>,
'processed_delta_model': <cosipy.image_deconvolution.allskyimage.AllSkyImageModel at 0x4076377f0>,
'background_normalization': {'albedo': 0.9969525475454646},
'alpha': 1.0,
'loglikelihood': [-1119047.9797920918]},
{'iteration': 29,
'model': <cosipy.image_deconvolution.allskyimage.AllSkyImageModel at 0x40768e200>,
'delta_model': <cosipy.image_deconvolution.allskyimage.AllSkyImageModel at 0x40768d600>,
'processed_delta_model': <cosipy.image_deconvolution.allskyimage.AllSkyImageModel at 0x40768e050>,
'background_normalization': {'albedo': 0.9969539225343819},
'alpha': 1.0,
'loglikelihood': [-1119000.7175890533]},
{'iteration': 30,
'model': <cosipy.image_deconvolution.allskyimage.AllSkyImageModel at 0x407637910>,
'delta_model': <cosipy.image_deconvolution.allskyimage.AllSkyImageModel at 0x407636620>,
'processed_delta_model': <cosipy.image_deconvolution.allskyimage.AllSkyImageModel at 0x407634310>,
'background_normalization': {'albedo': 0.9969552449401047},
'alpha': 1.0,
'loglikelihood': [-1118956.9770636673]}]
5. Analyze the results
Examples to see/analyze the results are shown below.
Log-likelihood
Plotting the log-likelihood vs the number of iterations
[15]:
x, y = [], []
for result in image_deconvolution.results:
x.append(result['iteration'])
y.append(result['loglikelihood'])
plt.plot(x, y)
plt.grid()
plt.xlabel("iteration")
plt.ylabel("loglikelihood")
plt.show()
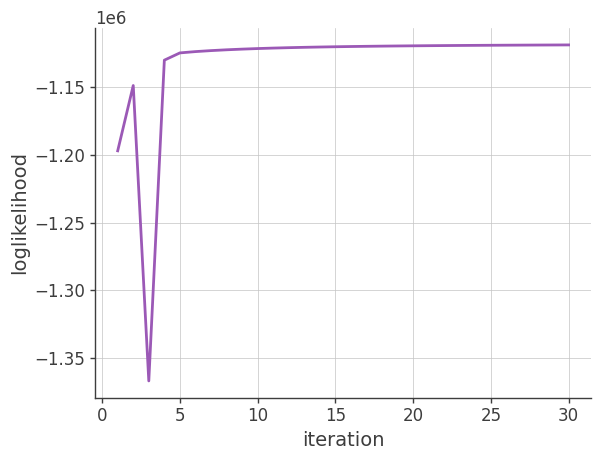
Alpha (the factor used for the acceleration)
Plotting \(\alpha\) vs the number of iterations. \(\alpha\) is a parameter to accelerate the EM algorithm (see the beginning of Section 4). If it is too large, reconstructed images may have artifacts.
[16]:
x, y = [], []
for result in image_deconvolution.results:
x.append(result['iteration'])
y.append(result['alpha'])
plt.plot(x, y)
plt.grid()
plt.xlabel("iteration")
plt.ylabel("alpha")
plt.show()
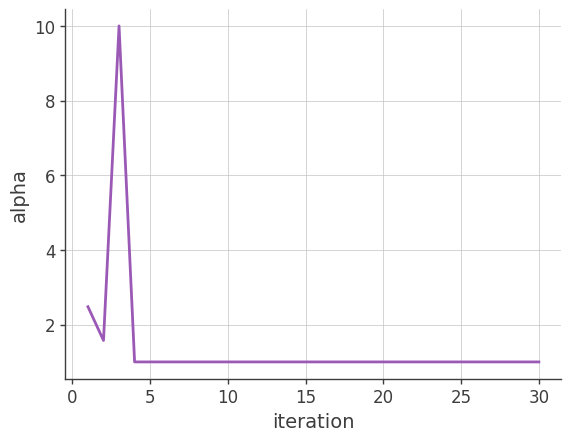
Background normalization
Plotting the background nomalization factor vs the number of iterations. If the background model is accurate and the image is reconstructed perfectly, this factor should be close to 1.
[17]:
x, y = [], []
for result in image_deconvolution.results:
x.append(result['iteration'])
y.append(result['background_normalization']['albedo'])
plt.plot(x, y)
plt.grid()
plt.xlabel("iteration")
plt.ylabel("background_normalization")
plt.show()
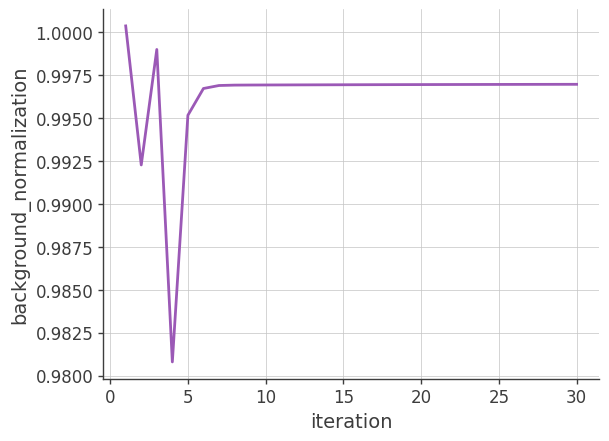
The reconstructed images
[18]:
def plot_reconstructed_image(result, source_position = None): # source_position should be (l,b) in degrees
iteration = result['iteration']
image = result['model']
for energy_index in range(image.axes['Ei'].nbins):
map_healpxmap = HealpixMap(data = image[:,energy_index], unit = image.unit)
_, ax = map_healpxmap.plot('mollview')
_.colorbar.set_label(str(image.unit))
if source_position is not None:
ax.scatter(source_position[0]*u.deg, source_position[1]*u.deg, transform=ax.get_transform('world'), color = 'red')
plt.title(label = f"iteration = {iteration}, energy_index = {energy_index} ({image.axes['Ei'].bounds[energy_index][0]}-{image.axes['Ei'].bounds[energy_index][1]})")
Plotting the reconstructed images in all of the energy bands at the 20th iteration
[19]:
iteration = 29
plot_reconstructed_image(image_deconvolution.results[iteration])
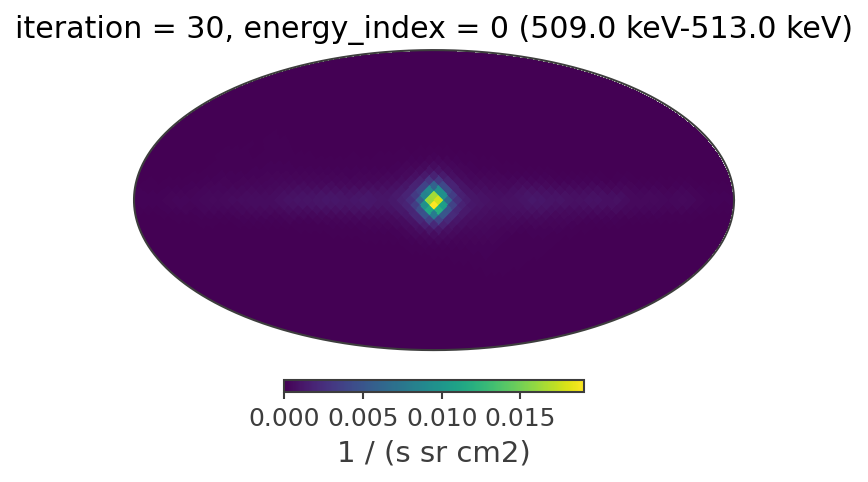
An example to plot the image in the log scale
[20]:
iteration_idx = 29
result = image_deconvolution.results[iteration_idx]
iteration = result['iteration']
image = result['model']
data = image[:,0]
data[data <= 0 * data.unit] = 1e-12 * data.unit
hp.mollview(data, min = 2e-5, norm ='log', unit = str(data.unit), title = f'511 keV image at {iteration}th iteration', cmap = 'magma')
plt.show()
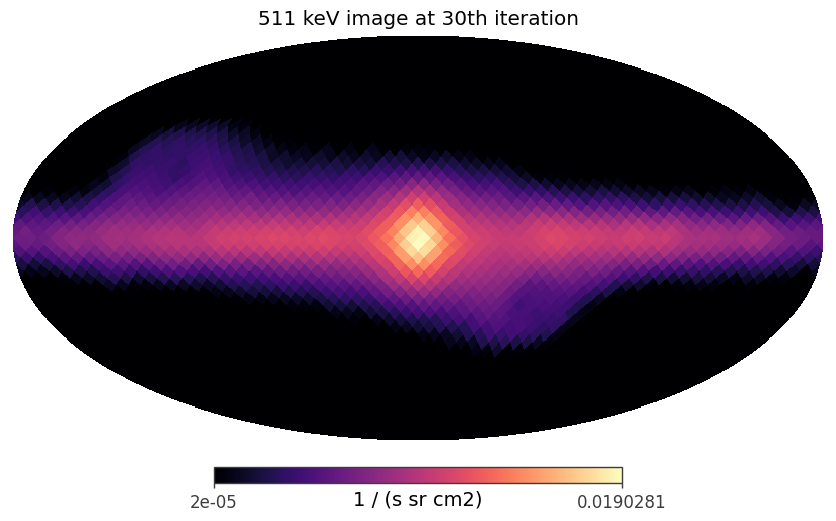
[ ]: